AI Detector Tools: Can They Really Tell Human from Machine?
- Andrew Perez
- Mar 31
- 9 min read
Naturally, one might question if the content we peruse is crafted by humans or produced by machines. This query isn't exclusive to us; it appears that 90% of enterprises harbor the same worry, concerned that AI-generated content could potentially damage their reputation if they fail to check for AI.
Delving into the intricacies of AI detection can help improve tools like Copyleaks that analyze text for AI usage. AI detection tools illuminates how these handy devices can provide reassurance. Stay with us to uncover more about accurate AI solutions!
Key Takeaways
AI detectors use machine learning and linguistic analysis to tell if content is by humans or machines. They learn from lots of data but sometimes make mistakes.
These tools often identify AI-generated content through various algorithms. AI detectors may wrongly flag non-native English writing, highlighting the need for advanced AI writing tools to enhance detection accuracy. as AI-made. This shows they need to improve and be fair to all writers.
False positives and negatives are big problems for AI detectors, affecting how well they find real human writing or machine writing.
New ways like watermarks in AI content and having experts check the work can help make sure content is original and truly written by people, and check for AI involvement.
The future of AI detecting will see better algorithms and working with other techs to more accurately spot AI-generated texts.
How Do AI Detector Tools Work?
AI detector tools work by using machine learning and linguistic analysis to distinguish between human-written content and AI-generated content. These tools also utilize probabilistic models to assess the likelihood of a piece of content being created by a human or a machine.
Machine learning and linguistic analysis
Machine learning and linguistic analysis play key roles in how AI detectors figure out if content is written by AI or humans. We use natural language processing (NLP), a kind of artificial intelligence, to understand and analyze human language, enhancing our basic AI capabilities.
This includes checking sentence structures, the way words are used together, and even the rhythm of the text. Machine learning algorithms learn from vast amounts of data to spot differences between human-written texts and those generated by AI.
Probabilistic models then predict how likely it is that a piece of content was created by an AI like GPT-4. These models look at patterns found during their training on large language models (LLMs) like GPT-3.5.
They get better with more data and can adjust as they learn from new examples of both human and machine writing styles, enhancing their AI score.
We harness technology to navigate the fine line between human creativity and artificial intelligence, particularly with major AI advancements.
Next up: we explore how AI writing detectors can improve accuracy. probabilistic models AI tools add another layer to this fascinating process of content verification, especially when using accurate AI detectors.
Use of probabilistic models
We use probabilistic models to figure out if content is made by AI or a person, our advanced AI detector helps clarify the source. These models look at how likely it is that certain words, phrases, and sentence structures come from humans or machines.
They learn from lots of data on human writing and AI-generated text. This way, they get better at telling the two apart.
For example, tools like OpenAI's detectors are trained with loads of texts from both people and AI systems. They use this training to make These tools make educated guesses about new pieces of writing based on their ability to detect AI.
By analyzing patterns and differences in language useThese tools can predict if an article was written by a machine, such as the Claude AI model.
Are AI Detectors Accurate?
AI detectors, including advanced AI models, aim to distinguish between human and machine-generated content, helping to identify if AI was used. They may encounter errors, including bias against non-native English writers, which can be detected by an AI checker. However, with advancements in detection algorithms, they hold promise for improvements in accuracy over time.
Common errors in detection
AI detectors sometimes get things wrong. They can mistake human-written text for content written by AI. This happens because AI relies on patterns in writing. But, not all human writing fits these patterns neatly.
We see problems too when AI checks work by people who don't write in English as their first language. These tools might flag their content more often when they analyze text for AI-generated indicators. It's not fair, but it shows that these systems need to improve.
Even the best AI tools make mistakes, showing there's room for growth and better understanding in how to detect AI.
Biases against non-native English writers
When assessing content from non-native English writers, AI detectors might show biases due to syntactic patterns, parts of speech, and language nuances that vary from standard English.
These biases might result in false positives in plagiarism checks and incorrect identification of AI-generated text as human-written. It's essential for business owners to acknowledge these limitations when using AI detection tools as they might unintentionally penalize non-native English writers based on linguistic variations rather than actual plagiarism or originality.
In addition, language complexities and AI tools can complicate communication. Cultural differences can affect how AI models interpret language and context. play a role in the challenges encountered by non-native English writers when their work undergoes AI content detection. This highlights the necessity for nuanced approaches and manual review processes that take into account the diversity of language use, particularly in academic settings where clarity is crucial, especially when using the best AI for content generation.
By recognizing these biases and exploring alternative methods such as manual content review by experts or customized training datasets that cover varied linguistic styles, business owners can aim for more inclusive and dependable content evaluation while upholding academic integrity.
Limitations of AI Detector Tools
AI detector tools have limitations. They may produce false positives and false negatives. Evolving AI-generated content, especially from models like Claude and Gemini, poses challenges for these tools.
False positives and false negatives
AI detectors sometimes make mistakes. False positives This can happen when the best AI detector wrongly identifies human-written content as machine-generated, affecting the overall AI score. On the other hand, false negatives Mistakes can occur when AI mistakenly categorizes machine-generated content as human-written, which highlights the need for effective content checkers.
These errors can affect the accuracy of plagiarism checkers and openAI’s text generators, potentially leading to misinformation and academic integrity issues.
It is crucial for business owners to understand these limitations in AI detector tools to make informed decisions about their use in detecting original content on websites and combating academic dishonesty.
By being aware of these challenges, businesses can explore alternative methods of detection such as watermarks in AI-generated content They may require an AI checker for ChatGPT or manual review by experts to ensure the authenticity of their online material.
Challenges with evolving AI-generated content
As business owners, we acknowledge the complexities in handling evolving AI-generated content. The continuous advancement of AI technology presents challenges in ensuring the accuracy and reliability of detecting machine-produced content from human-written material.
This presents a significant obstacle as AI detectors may struggle to differentiate between original human-created content and sophisticated AI-generated text, impacting plagiarism detection and academic integrity.
Moreover, the rise in deceptive techniques used by generators of AI content, including those created by Gemini, adds intricacy to this issue. As these generators evolve, they become more adept at imitating human language patterns, making it increasingly challenging for existing detector tools to effectively distinguish between authentic human-authored work and algorithmically generated text.
These emergent challenges require a constant reassessment of current detection methodologies within the artificial intelligence and natural language processing (NLP) realm, highlighting the need for ongoing research and development into more robust solutions.
Alternative Methods of Detection
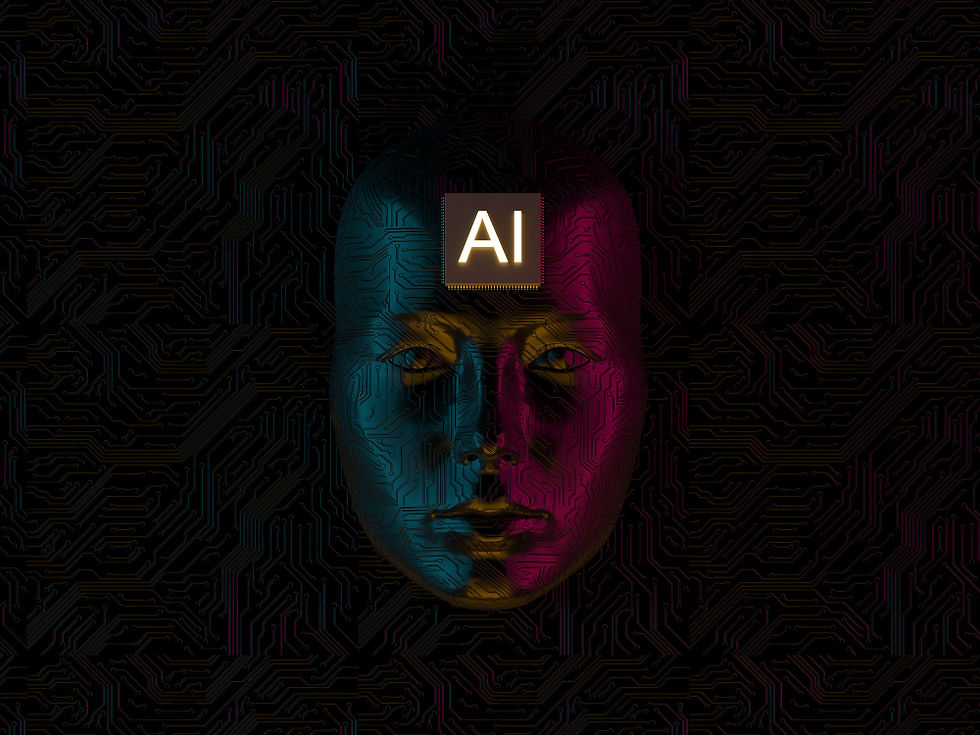
Consider examining AI-generated content for watermarks, and have experts manually review the materials to ensure their authenticity and originality, potentially using an AI checker.
Watermarks in AI-generated content
Watermarks in AI-generated content act as digital signatures AI tools that help identify the source or creator of the content are becoming increasingly important. Whether they are in the form of text, images, or invisible code embedded within the content, they play a crucial role in tracking and protecting original work in the expansive landscape of AI-generated content.
By incorporating watermarks, businesses can safeguard their intellectual property and maintain authenticity in a world where AI-powered tools are creating increasingly convincing replicas of human-created material.
It's vital for business owners to acknowledge the significance of watermarking their AI-generated content to prevent unauthorized use or replication by competitors or other entities.
This approach not only safeguards original work but also ensures that businesses retain ownership and control over their creations in an age where machines can replicate human-like thought processes with precision.
Including watermarks is an essential step toward securing and preserving the integrity of AI-generated material while also establishing clear lines of ownership and authorship.
Manual content review by experts
When ensuring content authenticity, it is crucial to check for AI to determine if AI was used. manual review by experts is essential. Human intelligence remains crucial in identifying nuances and contexts that AI detectors may overlook. This thorough examination offers an extra layer of assurance against misinformation and plagiarism, especially when dealing with complex or ambiguous instances of generated content.
Therefore, while AI detection tools play a significant role, the keen eye of human reviewers is vital for maintaining accuracy and quality.
The Future of AI Content Detection
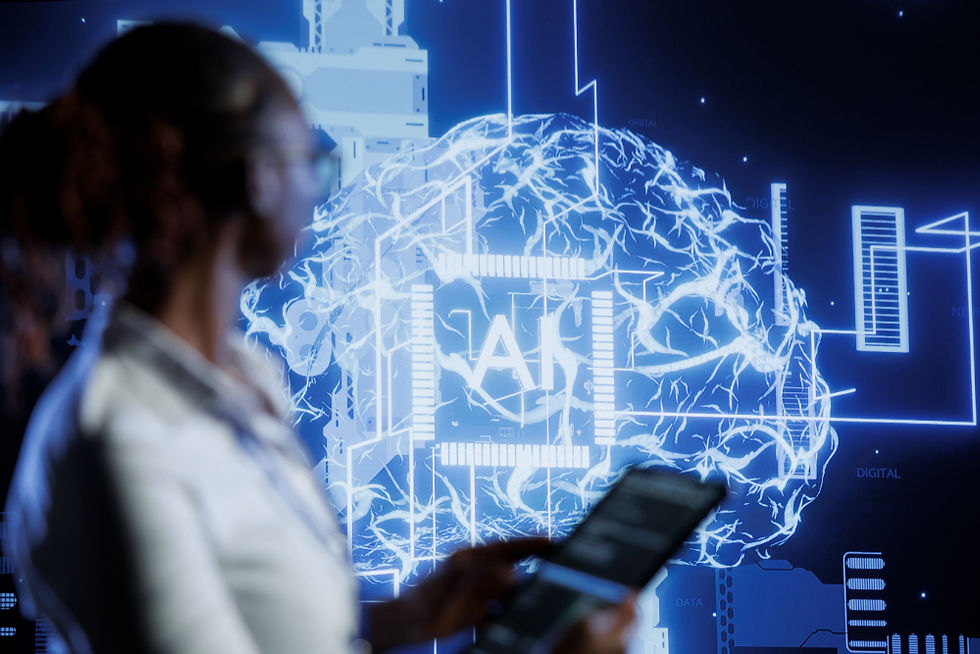
The future of AI content detection involves advancements in algorithms and the potential integration with other technologies. Improvements are tailored to enhance the reliability of AI detectors, particularly in the ever-evolving realm of AI-generated content and major AI developments.
Improvements in detection algorithms
Improvements in detection algorithms are essential for enhancing the accuracy of AI detectors. These advancements involve utilizing machine learning and linguistic analysis to refine the tools' capacity to differentiate between human-written and AI-generated content.
Moreover, integrating Unsupervised classifiers with decision trees and logistic regression are often employed in advanced AI systems. brings about more dependable detection results. Pairing statistical phrase structure analyses with predictive syntax enhances the precision of identifying AI-generated text.
As a result, these developments ensure that AI detectors can effectively counter misinformation, plagiarism, and academic dishonesty in digital content, particularly through the use of AI tools.
By including these advanced technologies into AI detector tools, businesses can better It is essential to safeguard their online presence against illegitimate or misleading content using free AI detectors.. The integration of these sophisticated algorithms significantly improves the reliability of detecting AI-generated material across various platforms and search engines while supporting efforts in maintaining originality and quality standards within digital content.
Potential integration with other technologies
After discussing the improvements in detection algorithms, it's crucial to explore potential integration with other technologies. We can create a more comprehensive solution by adding plagiarism detectors and grammar checkers to existing AI detector tools.
This combination will enhance the accuracy of detecting AI-generated content and human-written content, ultimately improving search engine optimization (SEO) and ensuring academic integrity.
Furthermore, integrating natural language processing (NLP) tools alongside AI detector systems can further refine the analysis of They analyze syntactic structures within texts to detect AI., reducing false positives and false negatives.
By leveraging machine learning (ML) techniques together with these technologies, we can elevate the reliability of AI content detectors in identifying original from generative AI-generated content while preventing academic dishonesty.
This approach aligns with the constantly changing nature of artificial intelligence and supports a proactive stance against misinformation propagation, particularly through advanced AI detectors.
In this complex domain of computer studies, embracing such integrations is essential for business owners seeking strong solutions to combat issues like self-plagiarism and fake news effectively.
Conclusion
In wrapping up, AI Detector Tools have potential but also limitations. They use Linguistic analysis and probabilistic models are essential components of advanced AI writing tools like Copyleaks. to detect AI-generated content. However, they often make errors with biases against non-native English writers.
Additionally, their reliability is hindered by false positives and false negatives.
As we look to the future of AI Content Detection, improvements in AI models are necessary for more reliable results. Integration with other AI tools could enhance accuracy further.
It's essential to consider alternative methods like watermarking and manual review by experts.
Ultimately, while AI detectors show promise in detecting machine-generated content, especially with the help of AI checkers, there's still a need for refinement and consideration of their limitations for accurate identification.
Explore how technology is shaping the workplace and discover opportunities in remote executive assistant jobs This is particularly useful for busy professionals who need an effective advanced AI checker for ChatGPT.
FAQs
1. What are AI detector tools and how do they work?
AI detector tools use machine learning, specifically large language models (LLMs) and natural language processing (NLP), to analyze content. They can tell if the content is human-written or generated by artificial intelligence.
2. Can AI detectors really distinguish between human-written content and ai-generated content?
Yes, AI detectors, such as the best AI tools, can often identify differences in text analysis that suggest whether a piece of writing was made by a human or an AI text generator like Generative AI.
3. How reliable are these AI detection tools?
While generally effective, some challenges remain for AI detection reliability such as false positives or cases where overfitting occurs in predictive analysis due to complexity or dimensionality issues within the data mining process.
4. Are there any applications of these detectors beyond distinguishing authorship?
Yes! Use our AI tools to detect AI-generated content. In addition to identifying ai hallucinations from chatbots, they're also used in academic integrity contexts like plagiarism detection to prevent academic dishonesty.
5. Could this technology help with misinformation prevention?
Absolutely! By being able to detect whether information came from humans or AIs like ChatGPT Plus and other AI models, it could play a significant role in preventing the spread of misleading information produced by generative AIs.
6. Does this technology apply only for English texts?
No, even though our examples are based on English texts; with labeled data available across languages and proper syntactic analyses done using techniques such as support vector machines or random forest algorithms; these technologies have potential applicability universally.
Comments